Three blog posts later, here we are. This Research Insights post is the final installment in our series on bid-ask spreads and their hidden volatility. Take a step back and remember where we’ve been.Â
Bid-Ask Spread Volatility Explained primed the pump with a case study, showing an individual contract anomaly impacting an iron condor on TLT.
Bid-Ask Spread Expectations laid the groundwork, showing traders what to expect in the bid-ask spread of their positions.
Bid-Ask Spread Anomalies: Risk & Opportunity explored the individuality of options contracts and how each contract has a unique potential for anomalies.
In those articles, we found that volatility in bid-ask spreads can create hidden and impactful pricing events. They are present at the tick-level of pricing, and a single contract anomaly can cripple an entire multi-leg position. While we did discover some basic clustering, we also learned that nearly identical contracts often have unique bid-ask spread volatility.Â
These findings, plus previously shared information on supply and demand, market makers, and other bid-ask spread influences leave us prepared for a final deep dive.Â
The Goal
We used terabytes of market data to answer five key research questions about bid-ask spread volatility and discussed how those answers can threaten or inform your trading decisions. Previous posts explained, defined, and clarified; we wanted to put that knowledge to work by exploring challenging questions in this final article.
After reading, you will have a more robust understanding of bid-ask spread volatility, allowing you to highlight specific concerns for your portfolio.
Methodology
Accurately answering these questions required a lot of data. We used a live NBBO data stream, collecting bid and ask ticks with a 500ms aggregation window. In other words, we received a tick update no more frequently than every half-second (approximately), although not every option contract updated that frequently.
We collected 16 days of market data from June 1 to July 1, with date selection or omission determined by data completeness and error rates. Our study included 13 underlying assets, with trading hours constrained to 9:45 - 15:45 ET to avoid volatility during price discovery. All timestamps provided are in Eastern Time (NYC) unless otherwise stated.
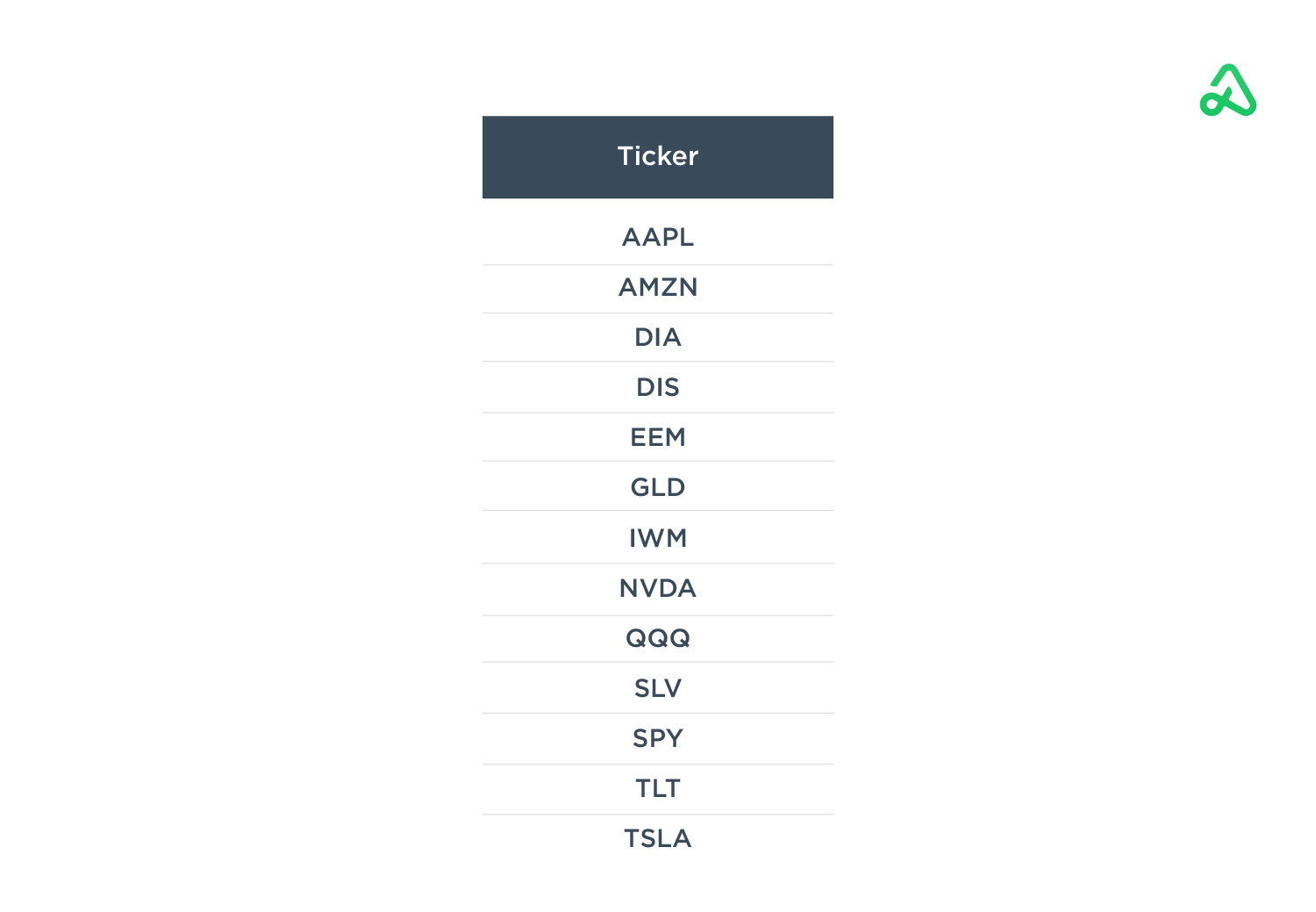
Heuristics and Statistics
To perform this study, we used heuristic and statistical methods. In basic terms, a heuristic is a “rule-of-thumb,” providing reasonable approximations using simple methods. Heuristics offered flexibility to efficiently answer meaningful questions with an acceptable level of confidence.Â
Heuristic One: We created a sliding 500-tick window, computing the average bid-ask spread. At any point in the trading day, if the next tick’s spread was greater than 2x the previous 500-tick average and also greater than 10% of the ask price, we recorded the event as an anomaly. Throughout this article, all mentions of anomalies conform to this definition unless otherwise stated.
Heuristic Two: Heuristic One did not provide information about the length of anomalies. To remedy this, we calculated the number of ticks it took for an anomalous data point to return to the 500-tick average spread. This method produced fewer data points than the first heuristic, as the bulk of data points were considered a continuation of previous events and therefore not recorded.
Our primary statistical metric was the coefficient of variation (CoV). This metric divides the standard deviation of the dataset by the mean, allowing for a standardized comparison across different underlyings.
Question 1: How Many Contracts Experience Anomalies, and What Level of Moneyness Is Most Susceptible?
Contract Participation
Knowing the percentage of contracts that had pricing anomalies was a natural starting point for this question and our overall study. We needed to know how many contracts had abnormalities when all other variables were stripped away before exploring theories.
Figure 2 provides this information, showing the average percent of contracts with an anomaly across all of our 16 collected days for each underlying. We generated this chart using Heuristic One, our anomaly detection heuristic.
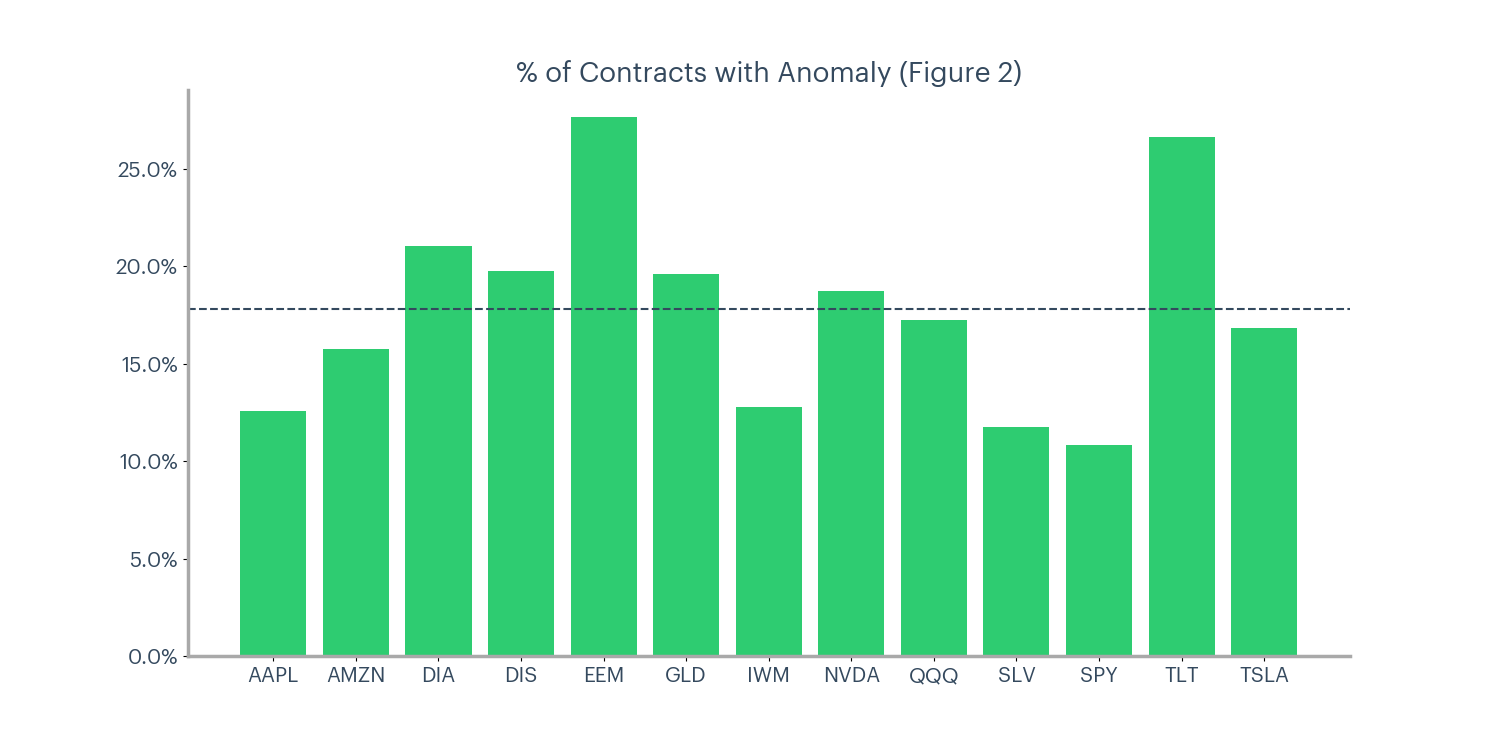
We found that, on an average day, 18% of contracts had an anomaly (shown by the dashed line in Figure 2).Â
Knowing that one in five contracts had significant bid-ask spread volatility was valuable, but didn’t offer practical improvements for trading systems. To enhance trader decision-making, we needed to know which contracts are most susceptible to these pricing anomalies.
In-the-Money vs. Out-of-the-Money
Having established base rates, we explored whether moneyness impacted the potential for anomalies.
Options contracts are more nuanced than simply in-the-money or out-of-the-money. To embrace this, we plotted a frequency distribution with the percentage ITM (positive) and OTM (negative) on the x-axis and the number of anomalies on the y-axis. Calls and puts are plotted in blue and green, respectively.Â
The underlyings’ closing price determined moneyness on each observation day, and we grouped data points based on their percentage ITM or OTM. For example, the AAPL 11 June 139 put contract was 10% OTM on June 2, and the AAPL 20 August 145 put contract was 10% OTM on June 18. The -10% group included the anomalous ticks for both these contracts on their respective dates.


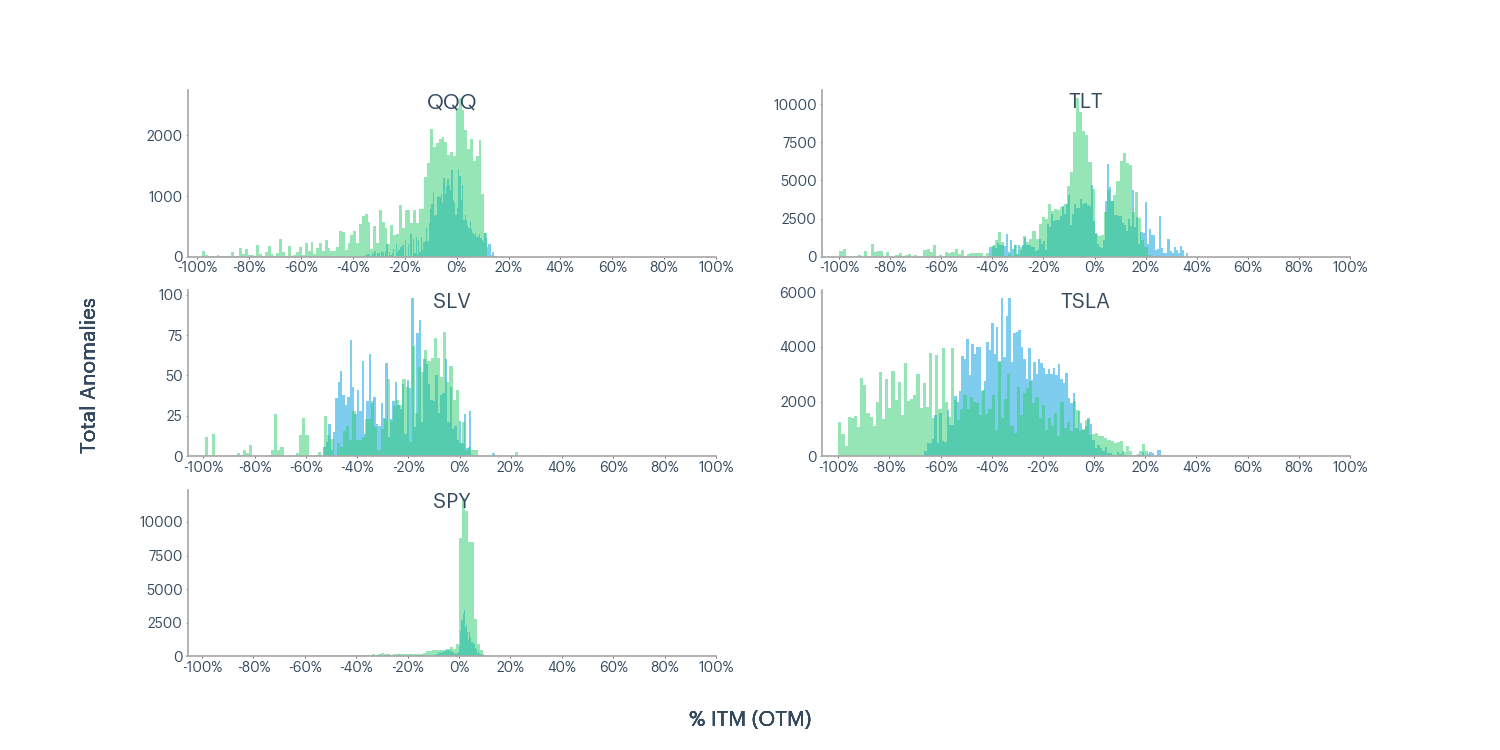
As evidenced by the overall left-skew of the distributions, we saw a visible bias toward out-of-the-money contracts, especially in puts further into the left-tail. In other words, Figure 3 suggests that OTM contracts have a higher prevalence of volatile bid-ask spread events. This finding was not meaningful until we tested a simple explanation.
If OTM puts had a higher tick-update frequency (i.e., If we collected more tick updates for OTM contracts), there would’ve been more opportunities for a tick to fit the anomaly definition, skewing our results. We tested whether this potential higher update frequency explained the left-skew in Figure 3.
If this simple explanation were true, we expected to see visibily similar distributions for tick frequency and total anomalies. To check whether this was the case, we calculated the average ticks per minute for every contract for each day of our study, then plotted each contract’s moneyness percentage on the x-axis and the contract’s ticks per minute on the y-axis. Each contract has 16 data points in Figure 4, one for each day in our study. Each plot shares the same y-axis scale.
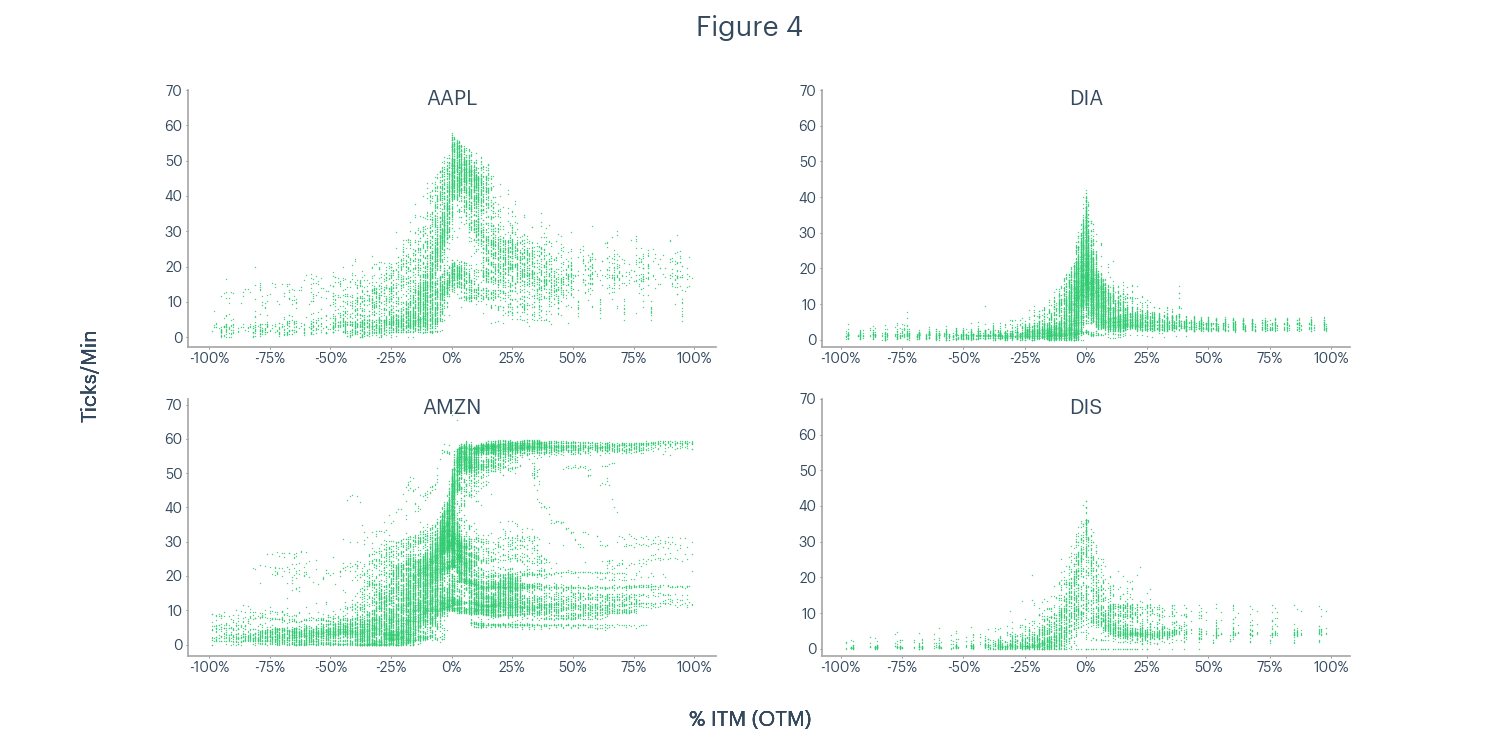

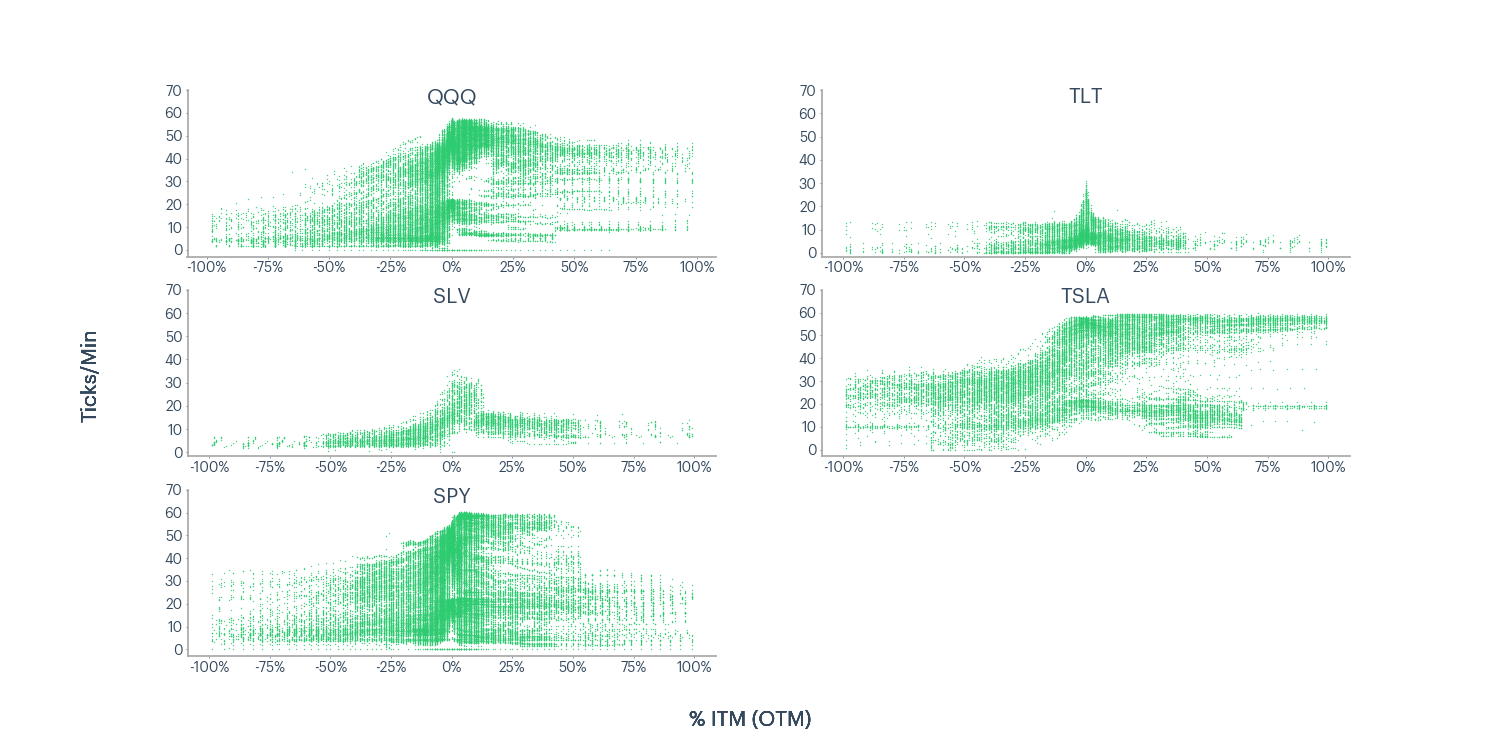
To confirm this simple explanation, we needed to see a left-skew in Figure 4, similar to figure 3. Instead, we found normal distributions for most underlyings and some with a higher update frequency for ITM contracts (right-skew). This finding confirmed that update frequency was not causing the outsized anomaly counts in OTM contracts. Therefore, there is strong evidence that OTM options, especially puts, have genuine price risk.
After analyzing the prevalence of anomalies across the option chain, we wanted to understand whether the magnitude (i.e., width of the spread) changed based on a contract’s moneyness.Â
Instead of plotting the number of anomalies and moneyness levels, Figure 5 compares moneyness levels with the average spread width of the anomalies. Calls and puts are plotted in blue and green, respectively.
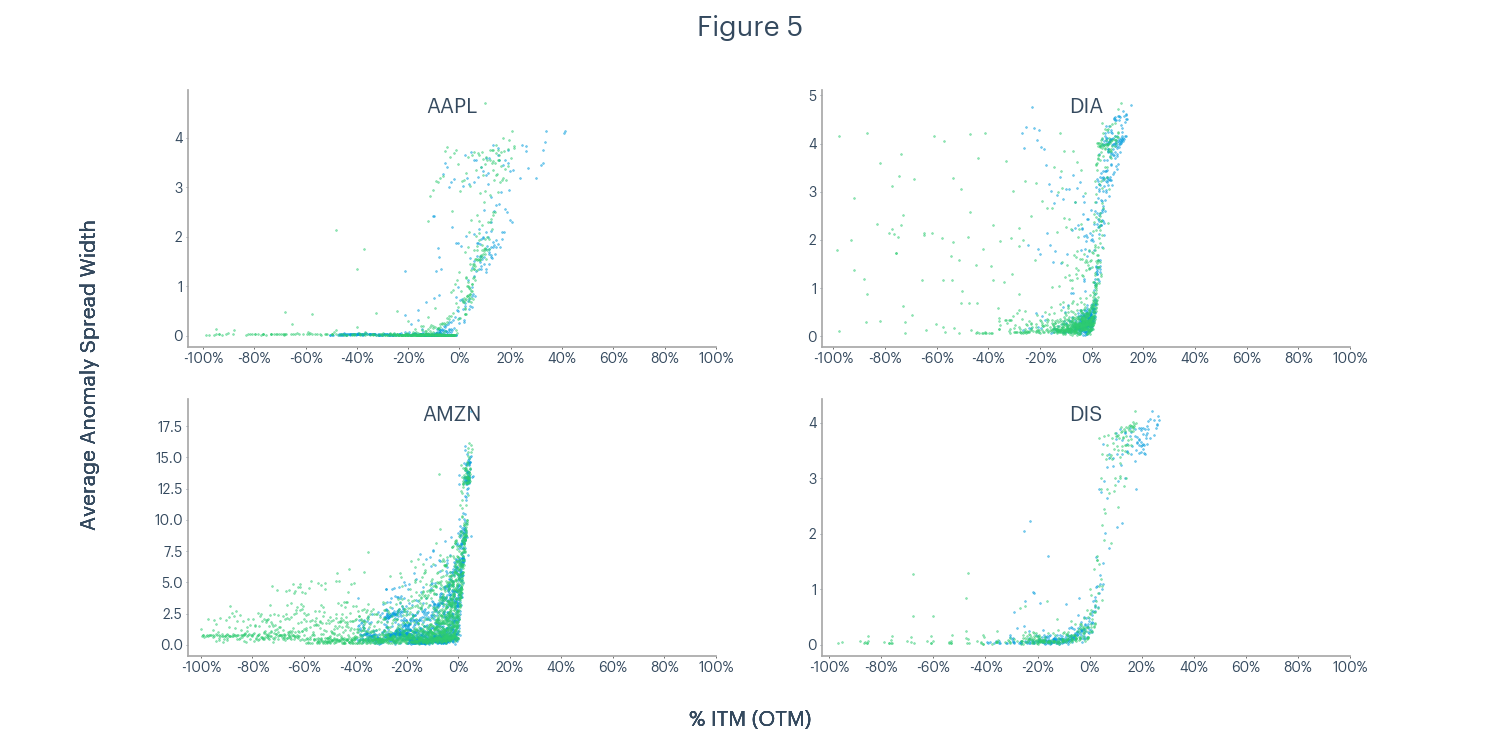
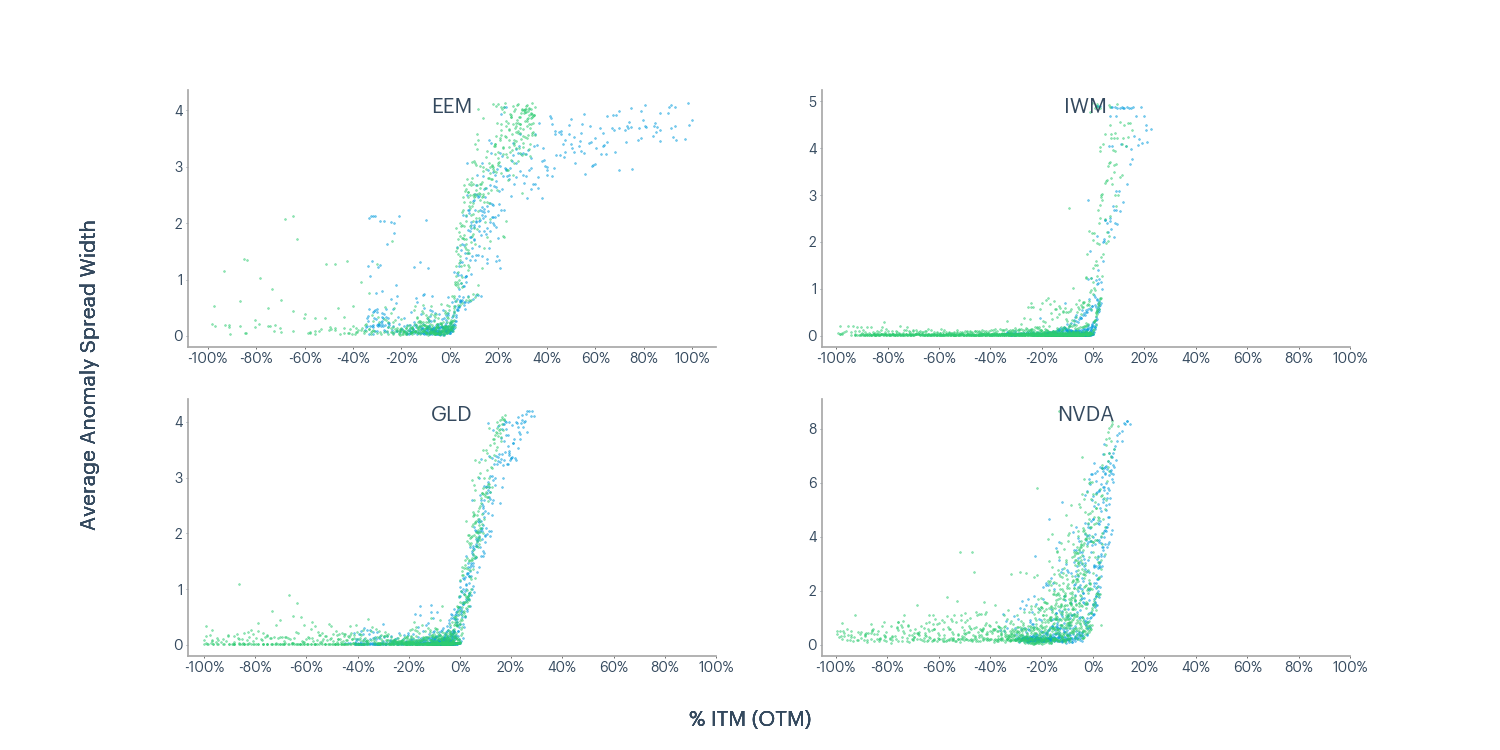
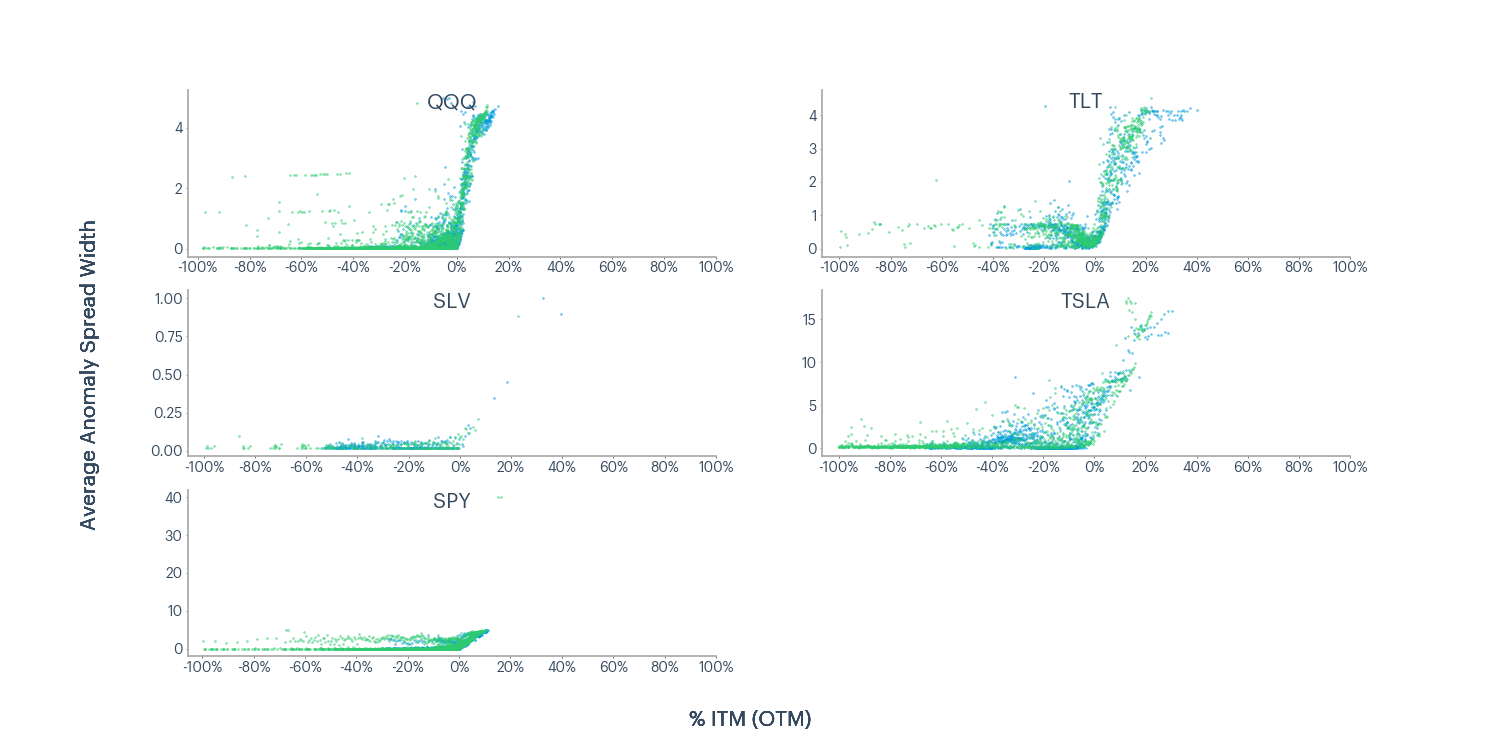
This analysis showed that pricing events ATM and ITM are more extreme than further OTM events, and it visually reinforced our earlier observation of put-contract dominance in OTM contracts.Â
Key TakeawayÂ
Traders should be aware of the above-average potential for pricing anomalies in OTM contracts, specifically puts. However, ATM and ITM contracts are uniquely risky given their larger magnitude pricing events. Something happens “behind the curtain” when contracts reach moneyness, creating higher magnitude anomalies.
To minimize the impact of these events, bot traders should include bid-ask spread filters in their automation decision tree before opening or closing a position. Click traders should apply a mental filter, questioning whether the spread looks typical for the type of contract and the specific underlying, and always use a limit price when placing options orders. Without these protections, an anomaly in a single leg could ruin the entire multi-legged strategy (as seen in the TLT case study).Â
Beyond this, traders should have safeguards in place for if/when contracts reach moneyness. Depending on the goal, they may exit the position or tighten the bid-ask filter to ensure a profitable exit price.
Question 2: Are Certain Expiration Types More Vulnerable To Anomalies, And Does Time To Expiration Influence The Prevalence?
We explored our data in two ways to understand the relationship between pricing anomalies and contract expiration type and length. First, we compared weekly and monthly expirations, then we aggregated the data by time to expiration.
Weekly and Monthly Expirations
To accurately report the number of weekly and monthly anomalies, we used per contract metrics. Generally, underlyings have more weekly contracts than monthly, and simply comparing total anomaly numbers would be misleading.Â
Weekly expirations often have lower liquidity than monthlies (i.e., weeklies are more thinly traded, with less volume and open interest overall), so we expected to see more pricing anomalies in these contracts.
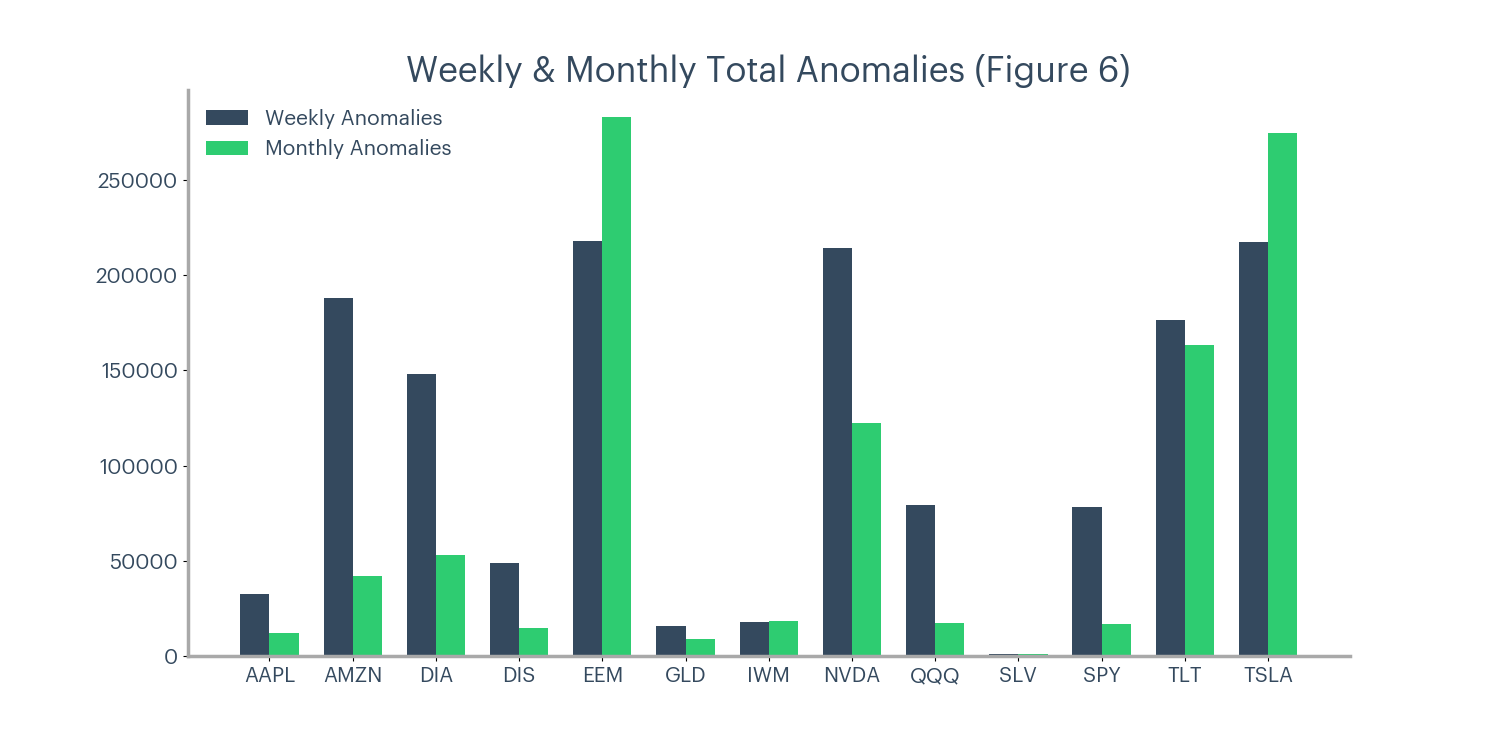
We were wrong. The results in Figure 6 show that expiration type does not impact the prevalence of bid-ask spread volatility as defined by Heuristic One. This conclusion held after standardizing for tick update frequency, as the mean difference between ticks per minute for weekly and monthly contracts was only 10%.
Underlyings have unique relationships between their weekly and monthly expirations, but our analysis found no cross-asset pattern. With a conclusive “no” on the question of a prevalence relationship, we decided to study the potential connection between expiration type and anomaly magnitude.
Figure 7 compares the average spread of anomalous events for all weekly and monthly expirations. It’s important to note that we were not comparing the overall average spread but only looking at the average spread for the recorded anomalous events.
To standardize for cross-ticker comparisons, we plotted the percentage difference between the weekly and monthly spread averages. A positive number meant the weekly contracts’ average bid-ask spread was X% greater than the monthly contracts’ average spread.

Our results showed that weekly contracts had a substantially higher average spread width when volatile pricing events occur. This divergence was not due to outliers, as confirmed by the median values overlaid in Figure 7. There are divergences between the mean and median, but choosing either value did not change the above conclusion.
Traders expect lower liquidity from weekly contracts, and wider bid-ask spreads often come as a result. You already know that. This finding is more profound. Despite the similar prevalence of pricing anomalies, we found that weekly contracts showed 69% (averaged across tickers) wider spreads during volatile pricing events.Â
Summarizing the conclusions from Question 1 with these findings, we find that traders should expect pricing anomalies across a broad range of OTM contracts, but the main risk for your orders and positions comes from ATM and ITM weekly contracts. We then wondered if a contract’s time to expiration added additional context to the previous findings.Â
Time to Expiration
To analyze whether the time to expiration impacted our findings, we grouped the data created with Heuristic 1 by days to expiration (DTE). We plotted each DTE value and the average spread width for the group. For example, both the SPY 7 July 409 put and SPY 23 June 413 put had five DTE at certain points of our study. The ticks recorded while each contract had five DTE were added to the same group.


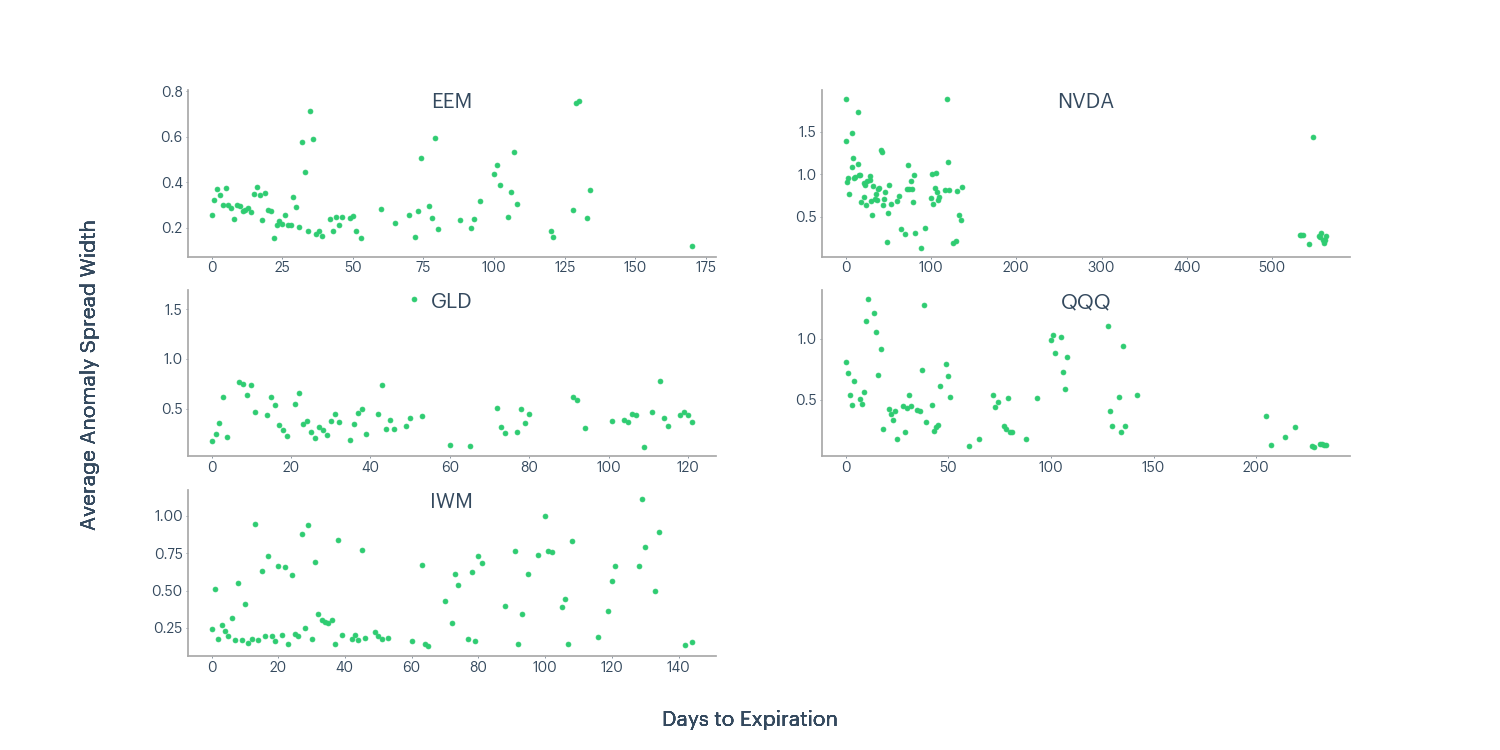
This analysis showed no evidence that the magnitude of a spread anomaly was related to the DTE of the contract. If that relationship existed, we expected to see either an upward sloping trend (positive relationship) or a downward trend (inverse relationship) across underlyings. Figure 8 demonstrates neither.
Further analysis (not pictured) did show an inverse relationship between DTE and anomaly prevalence, but this is likely due to the higher market participation in closer to expiration contracts. As mentioned before, a higher tick update frequency creates more opportunities to discover pricing anomalies.Â
Key Takeaway
Weekly and monthly expirations have similar anomaly prevalence, but weekly contract anomalies have a much higher magnitude. Time to expiration has no observable effect on the magnitude of pricing volatility.
When managing trades, remain aware of the increased risk in weekly contracts. The higher magnitude events are more likely to “shake” you out of a position by hitting a stop-loss. Increase the range of your bid-ask filters, and don’t forget the double risk of a weekly contract crossing into the money while holding. Or, most extremely, trade your strategies without weekly contracts. That and all the other suggestions are easily applied in automated trading, as shown in this tutorial.
Question 3: Are There Observable Patterns In Anomaly Occurrence?
Users in the Community have shared concerns that odd pricing events occur at regular intervals, such as the quarter hours. Since our bot automations run on 15-minute increments, this concern is front of mind for our users and the platform team at Option Alpha. We leveraged our data to test this clustering theory and observed whether or not anomalies were more likely to occur at specific times.
We grouped our anomaly data from Heuristic One by rounding down to its minute of occurrence across all observed trading days. Pricing updates that occurred at 10:01:15 or 13:01:27, for example, were added into the one-minute group. We plotted the percent of total anomalies for each minute group in Figure 9. The mini-charts have the same x and y-axis scale.
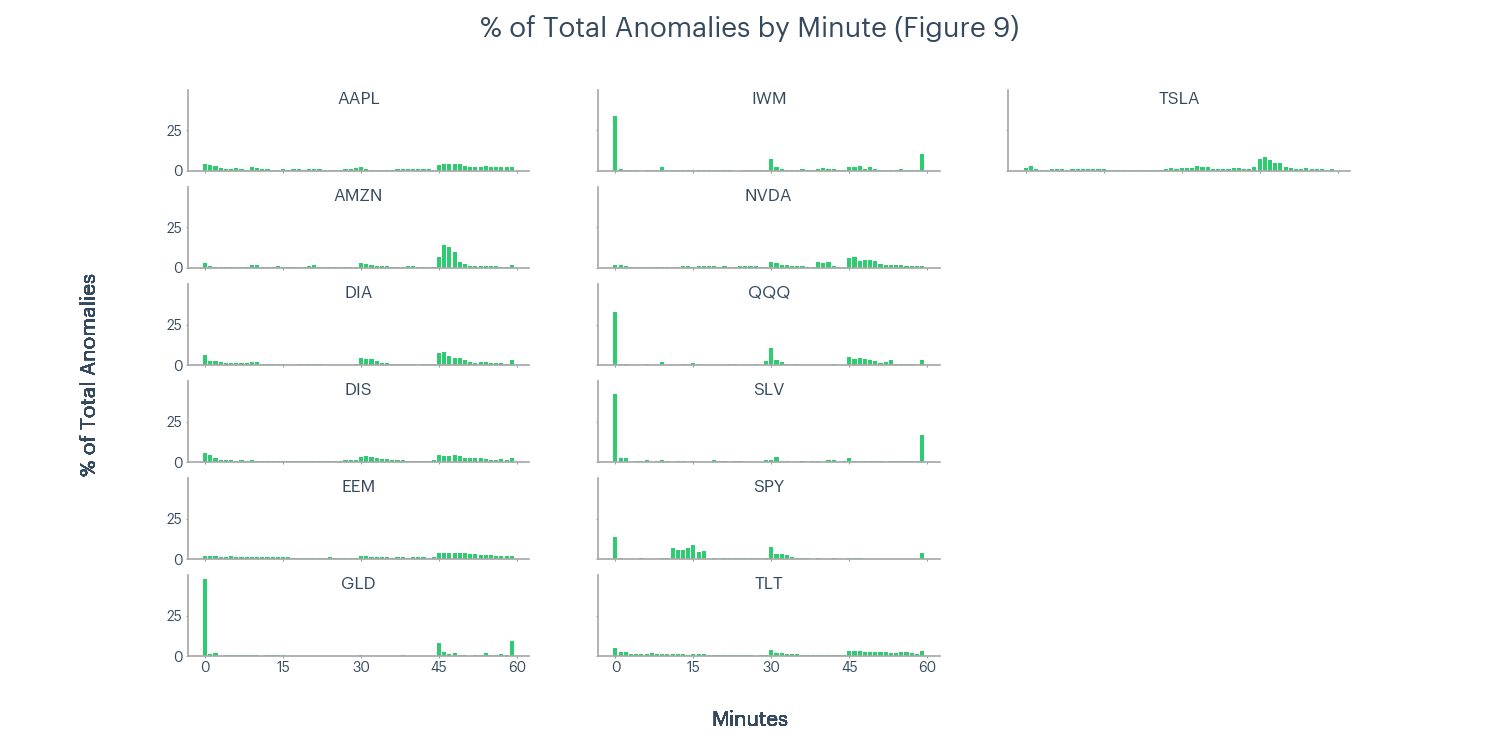
We found heavy clustering in specific tickers at the 0-minute market (i.e., the beginning of the hour). On average, this bar was 66% comprised of 10:00 AM observations, meaning more than half of our anomalies on the hour mark occurred at 10 AM. We are not sure why this heavy clustering occurs.Â
This phenomenon only occurred in four tickers and on 6 of 16 days. On June 1, we saw 73% of total GLD anomalies occurring between 10:00:00 - 10:00:59, while later dates showed 0 anomalies in this one-minute range. These are seemingly random, unpredictable pricing events. We could not discover why volatile events cluster at 10 AM, but we did use our data to identify more regular anomaly hotspots.Â
Some of these clusters became more evident when we plotted the same data as in Figure 9, with data points from 10:00:00 - 10:00:59 excluded.
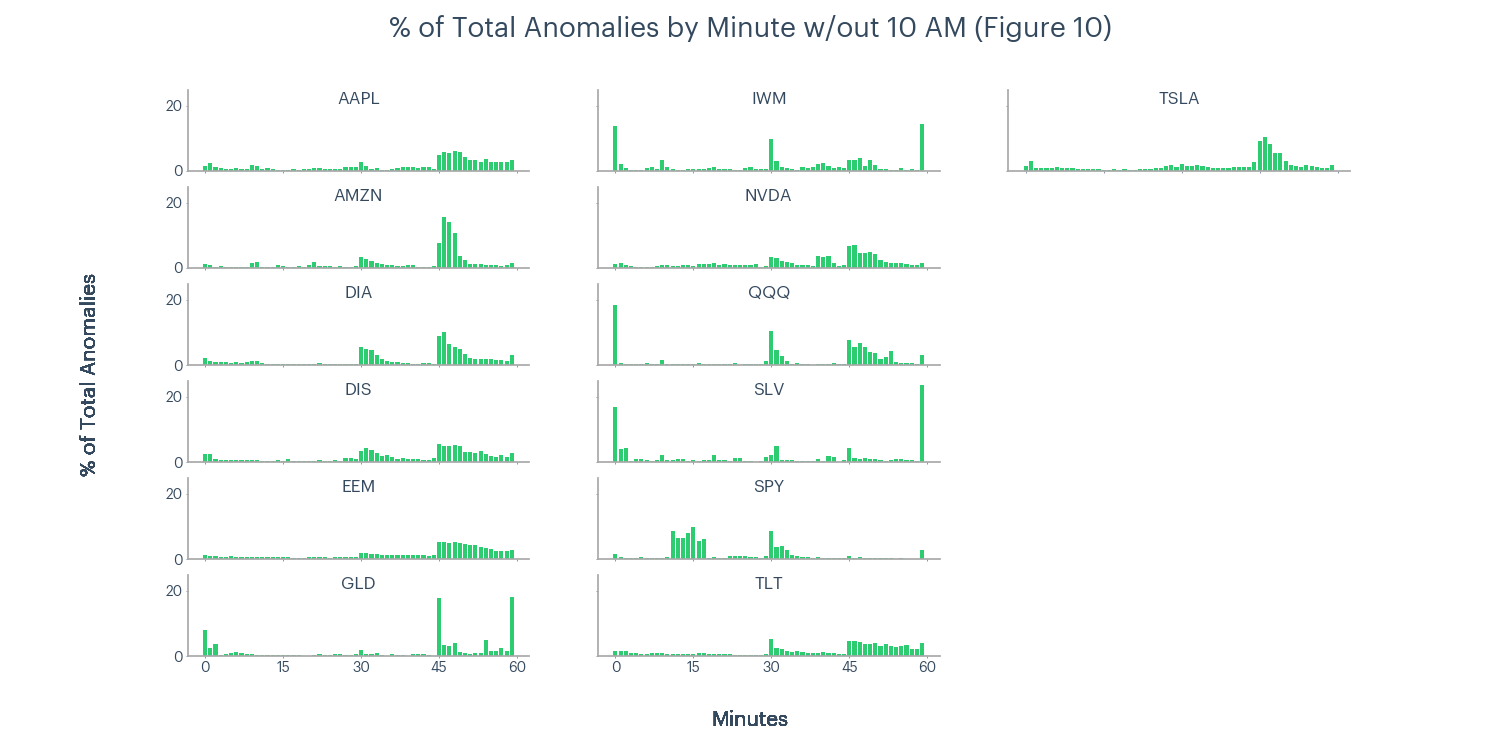
Our analysis showed results consistent with our users’ hypothesis. We saw clear visual evidence of events falling on 15-minute intervals and the surrounding times. We can append this information to our earlier findings. Traders face high bid-ask spread pricing risk when trading close to the money weekly contracts, and these events are most prevalent at regular 15-minute intervals.Â
Key Takeaway
Option Alpha users were correct that volatile pricing events often happen at regular times. We found heavy clustering at 10 AM and high prevalence on 15-minute marks (e.g., 10:00, 10:15, 10:30, 10:45) throughout the trading day.Â
The team at Option Alpha is researching this phenomenon to understand if and how it will impact the execution of automated trades. To prevent adverse market moves from affecting your fills, remember that automated does not mean unattended. One significant value of an automated trading system is how you can offload your mental checklists onto a bot, freeing up your time for strategy development. If you are handing this mental list to a bot, be sure you don’t forget any basic steps you took as a click trader, including checking the relative level of bid and ask prices.
Question 4: How Long Do Bid-Ask Spread Anomalies Persist, And Does The Magnitude Of An Anomaly Impact Its Persistence?
Using Heuristic Two, we found incredible variation in the length of anomalies. As a reminder, Heuristic Two helped measure the number of ticks an anomaly (as defined in Heuristic One) lasted. We saw event lengths ranging from 2 ticks to over one million.Â
Traditional mean values are deceptive and challenging to visualize given this wide skew, so Figure 11 shows the median anomaly length for each underlying’s interquartile range (IQR). The IQR contains the data between the 25th and 75th percentile. Using this adjusted dataset can improve analysis by removing the outliers on either side of the mean.
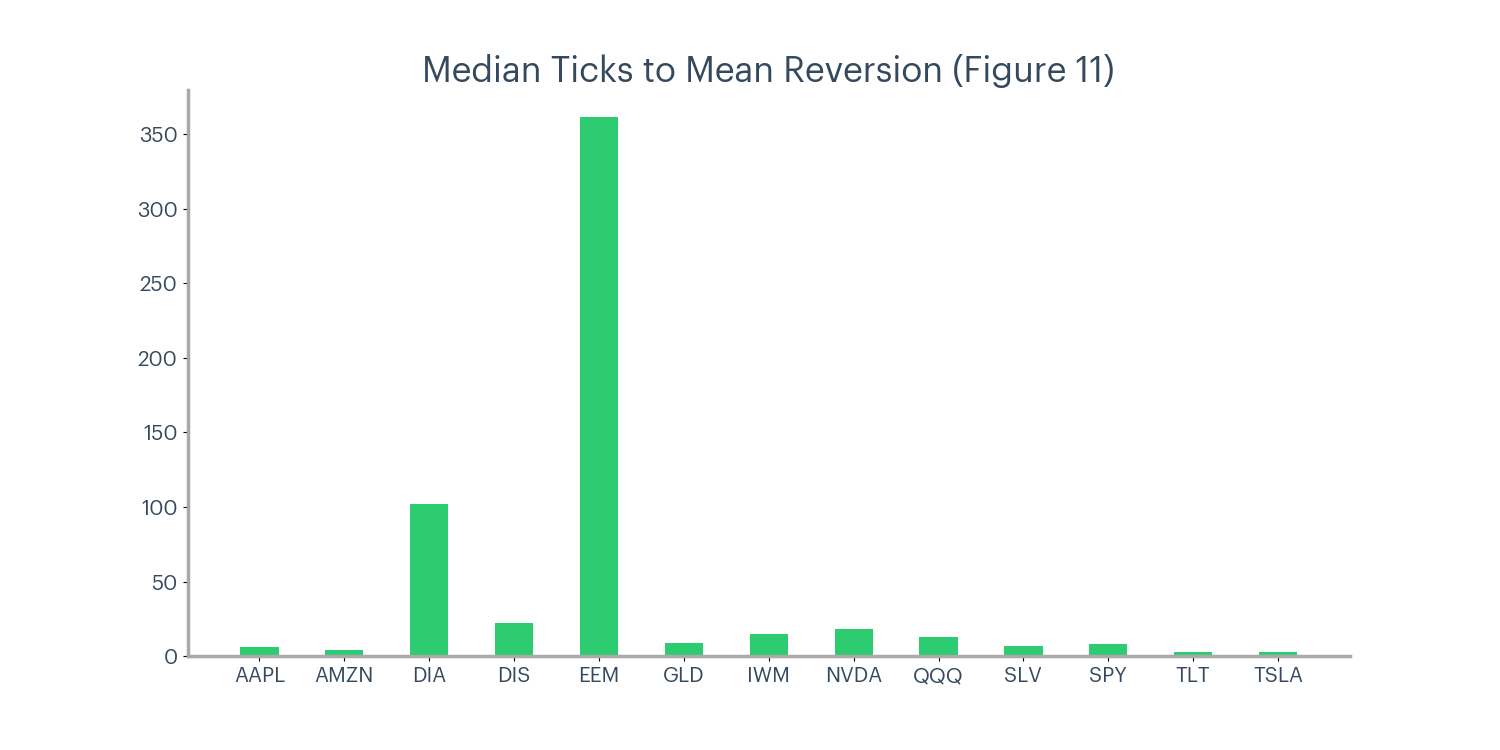
In the aggregate, these events were short-lived. For example, the typical SPY anomaly persisted for ten ticks, or 20 seconds, given our frequency data. Both DIA and EEM showed abnormally high median values but had an overall distribution similar to the other tickers.
Overall, we found no meaningful relationship between anomaly magnitude and persistence. To demonstrate, we generated the scatter plot shown in Figure 12, comparing spread as a percentage of ask price (magnitude) on the x-axis with anomaly length on the y-axis.

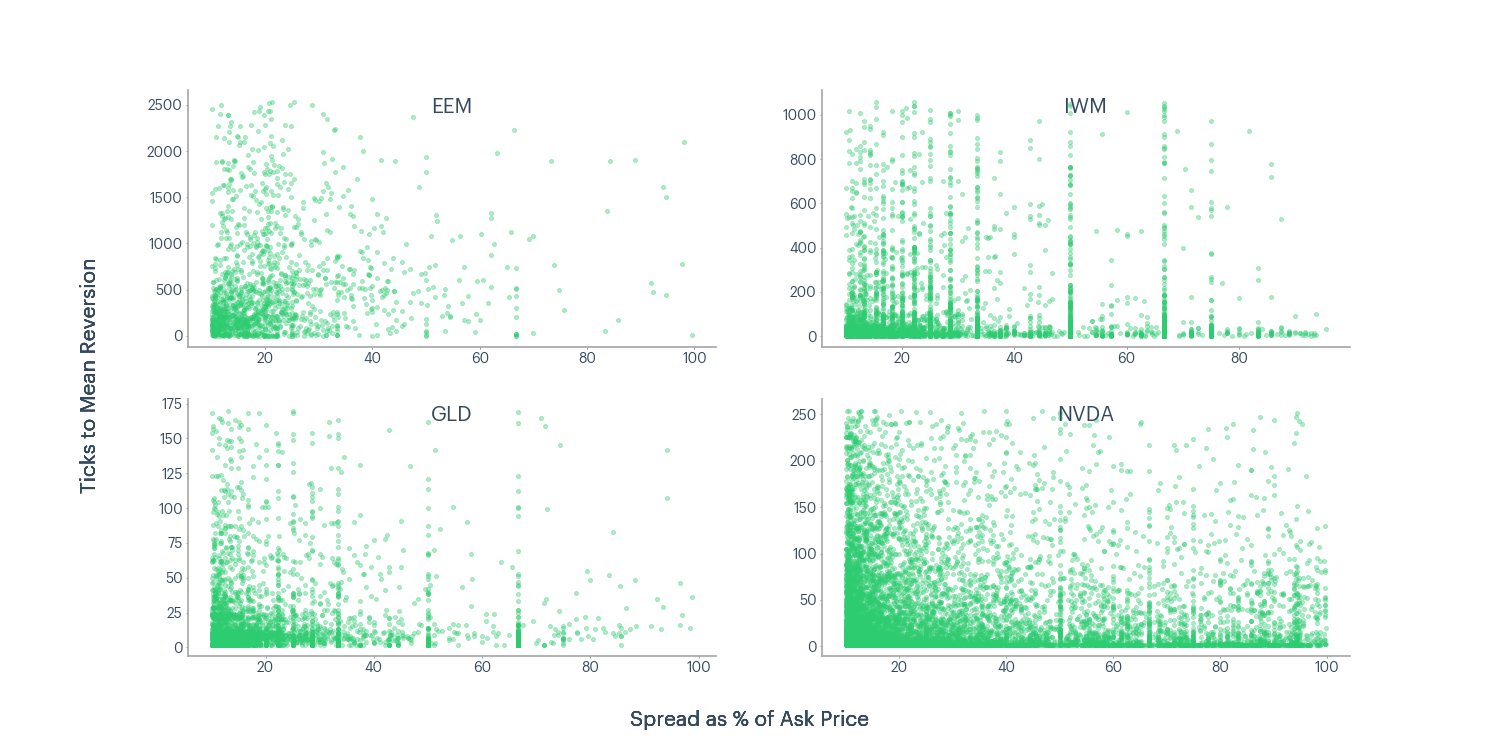
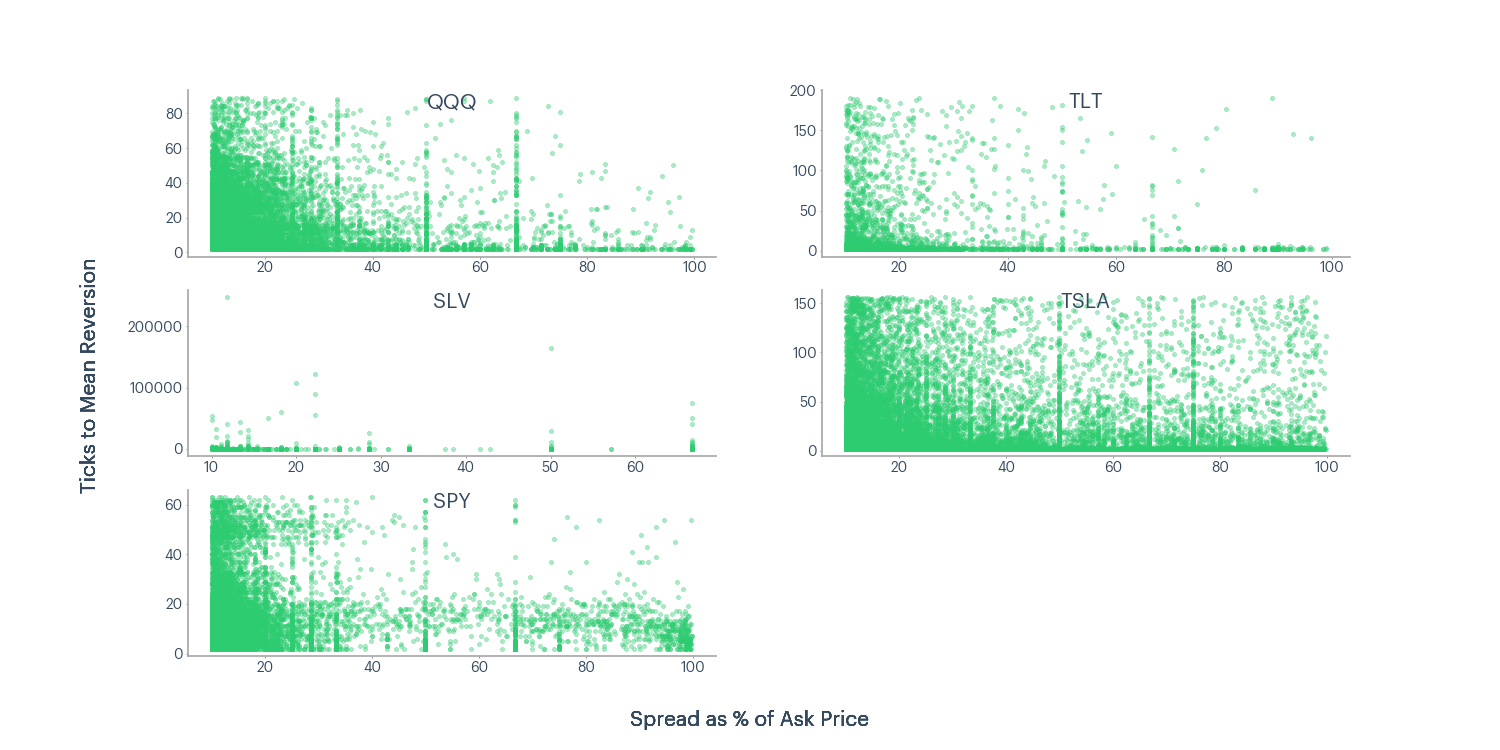
The left-skew in Figure 12 does not represent a relationship between anomaly magnitude and persistence. Instead, it simply demonstrates that our dataset contained more low-magnitude observations than high-magnitude. Because of our process in Heuristic One, this was an expected result.
To prove that anomaly magnitude influenced the event length, we needed to see a positive or negative trend on the y-axis (i.e., increasing or decreasing y-axis values as you move right in Figure 12). We did not see that trend or any other evidence supporting a relationship between magnitude and persistence.Â
Key Takeaway
The persistence of volatile bid-ask spread events differs greatly based on the underlying asset, but that tick-length is not directly related to the event’s magnitude. This is another relevant reminder that each tradable asset exhibits a unique “personality” within its market.
Question 5: Do Certain Assets Always Show More Volatile Bid-Ask Pricing, And How Does The Assumption Of Liquidity Correlate With That Behavior?
Our previous discussions have identified prevailing trends in spread behavior across the 13 studied underlying assets. For the final question, we compared each underlying to identify differences, not confirm a cross-asset hypothesis. To accomplish this, we branched away from our previous heuristic methods in favor of statistical calculations.Â
We calculated the coefficient of variation for each contract’s spread on each day. CoV measures the variability of a dataset with the standard deviation, then divides that value by the mean, allowing for comparison to other datasets. Figure 13 shows the mean and median CoV values for each underlying, along with the average ticks per minute.
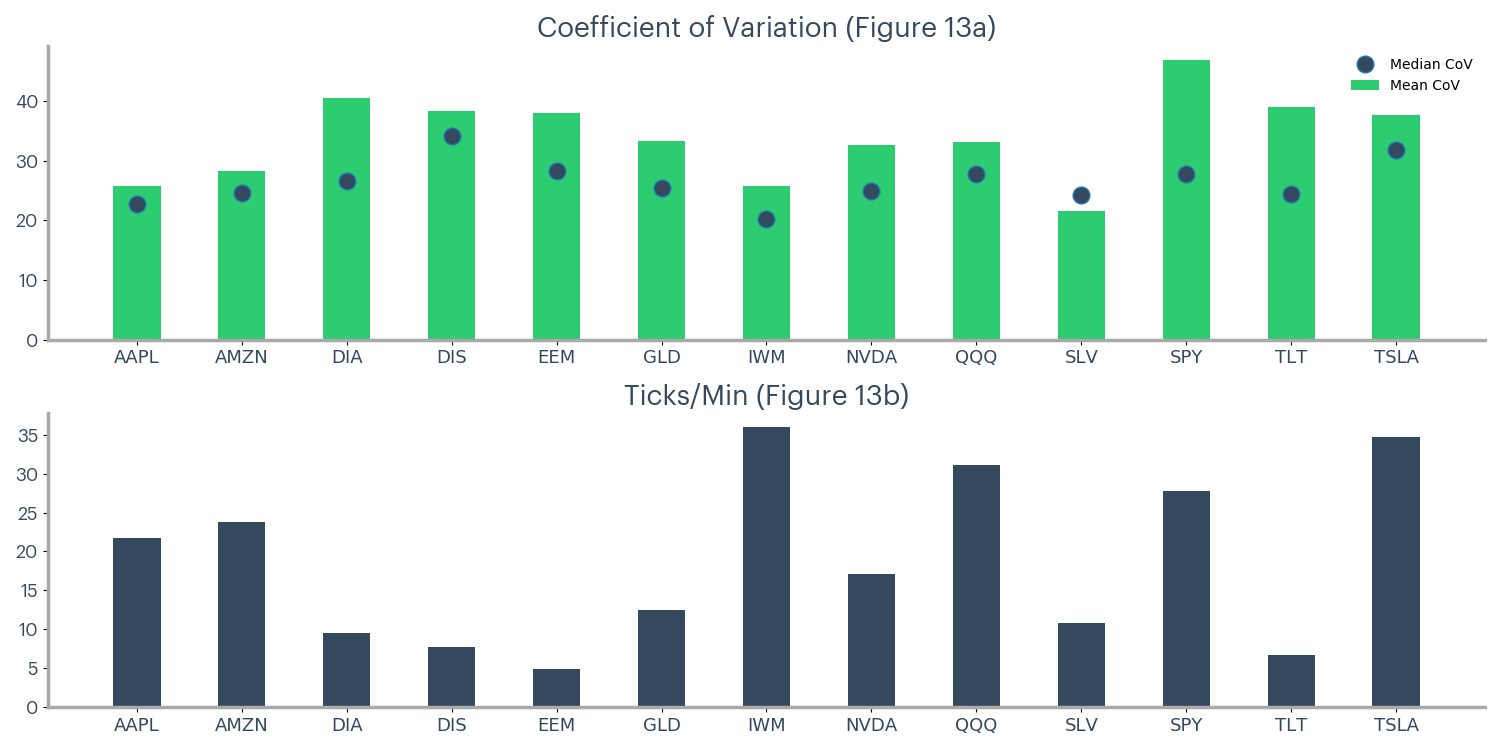
To answer Question 5, we explored the two panes of this chart individually. A deeper dive into the top pane answered whether specific underlylings are always more volatile, and pane two offered context on the impact of liquidity.Â
Underlying Variability
In its basic, summarized form, Figure 14a showed SPY with the highest variability and SLV with the lowest. However, we were unsure if those conclusions held across all the days in our study. The divergence between the mean and median values for SPY offered a hint. We generated Figures 14a and 14b to begin answering this question. We plotted the CoV across dates for the two highest and lowest averages from Figure 13a along with the average CoV for all tickers.
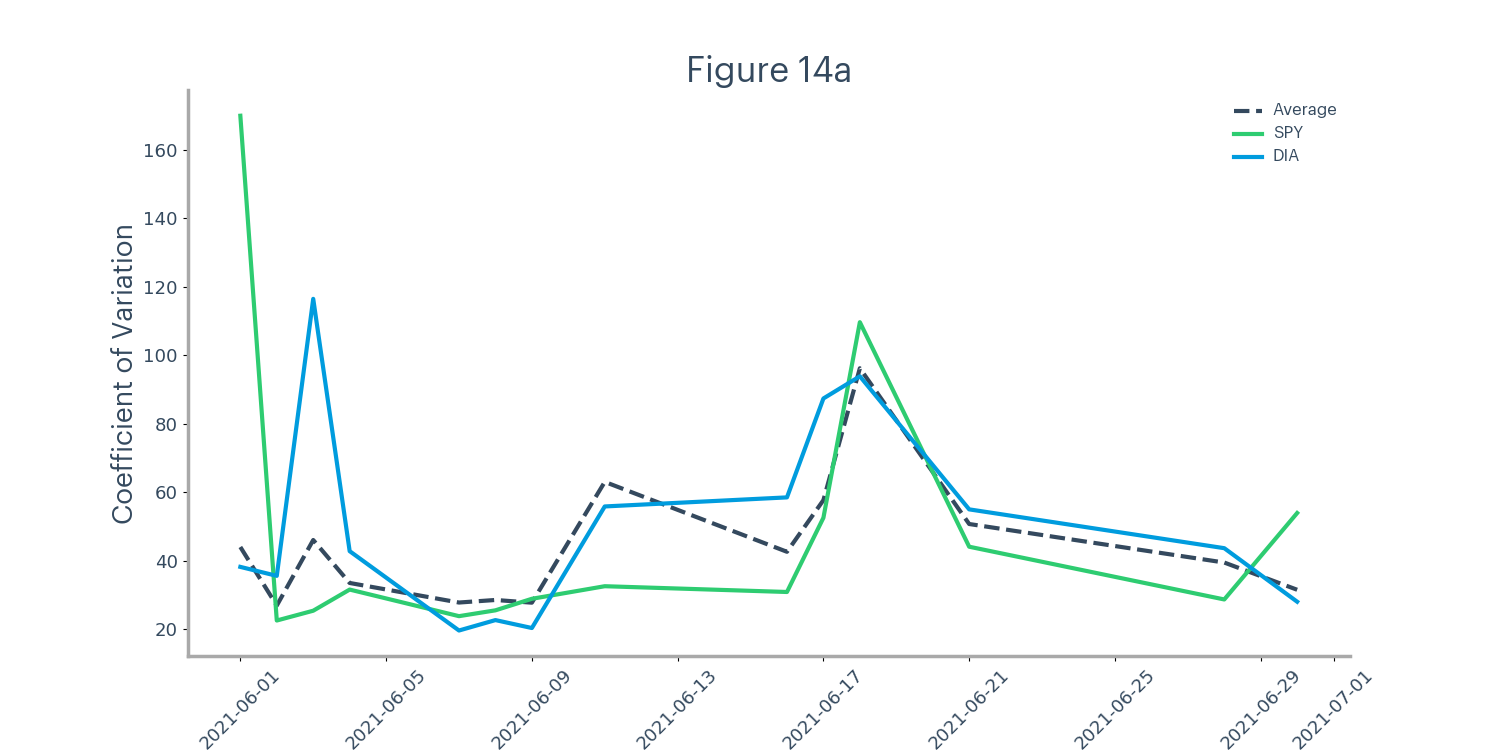
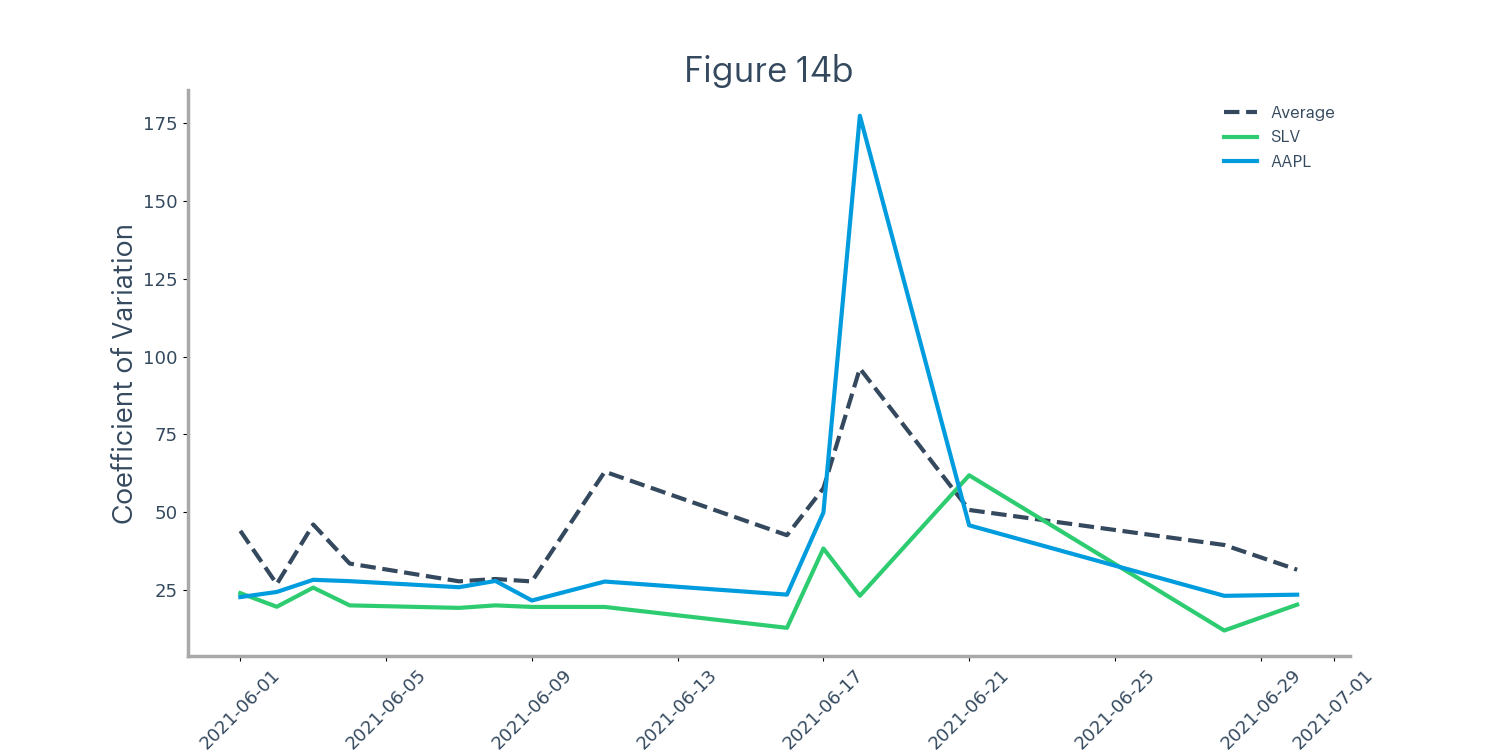
We immediately understood from Figure 14a that SPY did not show the highest variability across all dates. Yes, its overall value was highest on average, but now we know the reason for the mean and median divergence; June 1 was an unnaturally volatile day for SPY contract spreads. DIA was closer to showing sustained, above-average volatility across all studied days.
Conversely, SLV was well below the average CoV in Figure 14b for all days except June 21, supporting the idea that SLV generally shows low volatility in bid-ask pricing. The same is true for AAPL, which had below-average spread volatility for all days except June 18. Interestingly, on June 18, 10 of 13 underlyings showed a spike in spread volatility; AAPL followed the trend.
Overall, we identified two significant facts. First, certain tickers (e.g., SLV and AAPL) show sustained low bid-ask spread volatility relative to other assets. However, other tickers (e.g., SPY) may seem highly variable, but that observation does not persist across days. Secondly, we found that underlyings’ spread volatility correlates (e.g., mutual spike on June 18).Â
Role of Liquidity
Figure 14b provides data on the liquidity of each underlying asset. Most traders would assume lower liquidity produces more volatility, whether in the underlying price or the bid-ask spread. However, we concluded that SLV showed the lowest sustained volatility in our study, and SLV had below-average ticks per minute. Similarly, AAPL showed sustained low volatility and had an average ticks per minute value.
A possible explanation for this disconnect is the structure and operation of the electronic order book. A Level 2 view shows bids and asks with the number of contracts offered or requested. If in Figure 15, a group of bids consumed all the asks at $435.08 and $435.09, the spread would temporarily jump as the ask price moved to the next available $435.15
An underlying with more ticks (i.e., higher liquidity) has more chances for these jumps to happen, creating a perceived higher variability despite the high liquidity underlying.
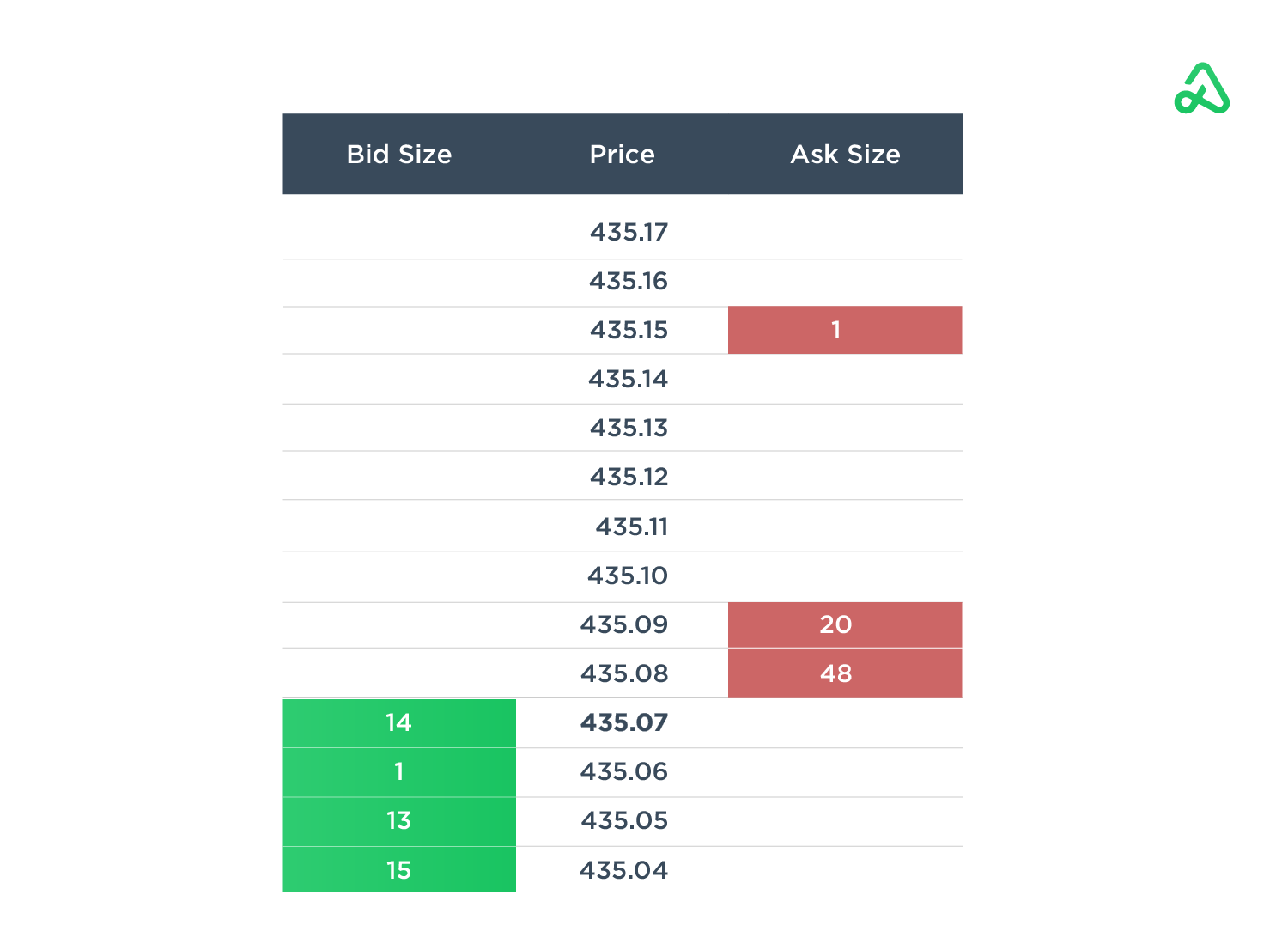
Key TakeawayÂ
SLV and AAPL have sustained low bid-ask spread variability, and DIA has a higher variability range than average. Our study found no evidence that these phenomena are created or influenced by the perception of liquidity. We expect that the electronic order book explains part of the disconnect between liquidity and variability.
As a general rule, no underlying asset is safe from bid-ask pricing volatility. Some, like SLV, may be less sensitive, but that does not free you from applying the risk management practices mentioned in previous sections. With the efficiency of bots and automated trading, you have nothing to lose in adding more guardrails.
Conclusion
The research presented in this series of articles provides evidence for the existence of extreme bid-ask spread volatility. This volatility typically presents as unpredictable, short-duration spread jumps, or anomalies. The underlying asset and individual contract attributes uniquely impact the prevalence and magnitude of these anomalies. These unique events can materially affect your order execution if you do not apply proper risk management.Â
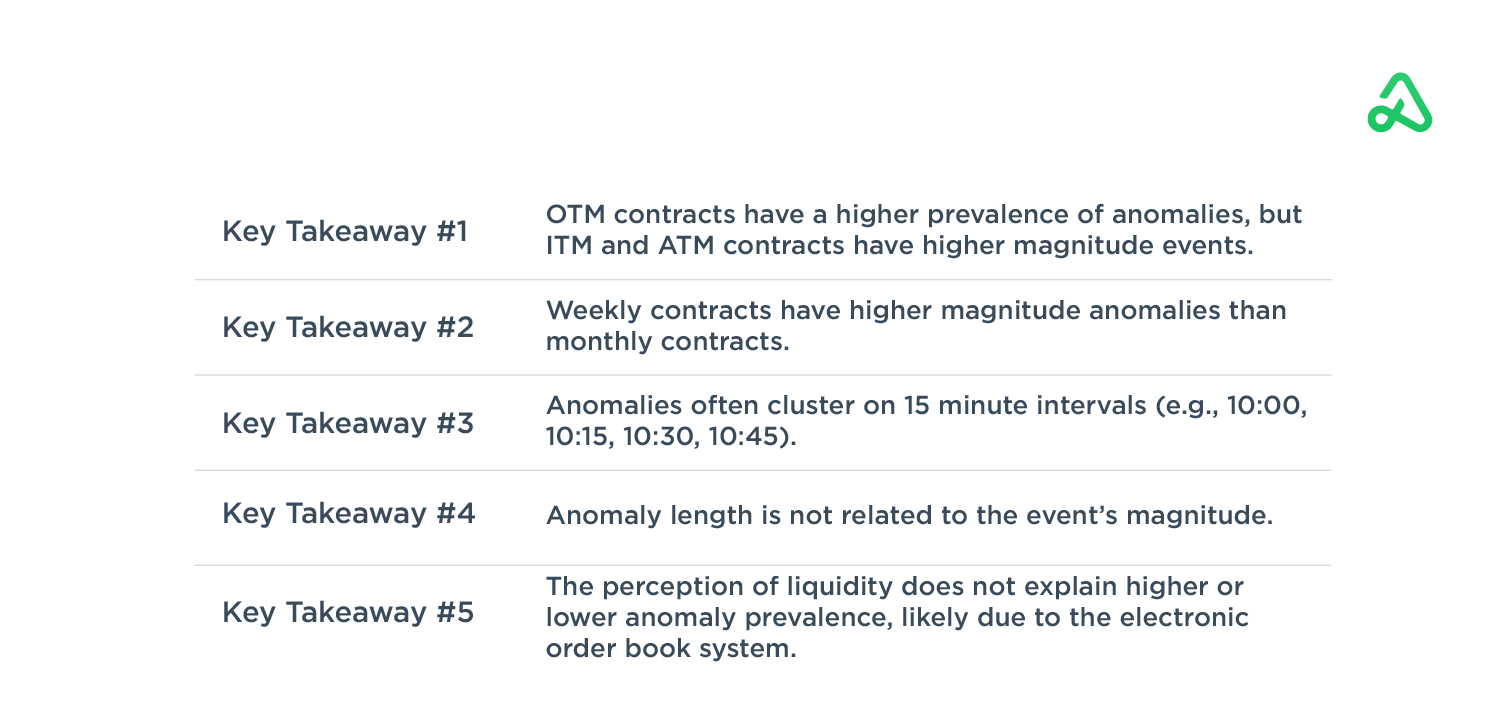
The key takeaways mentioned throughout this article not only alert you to the risk of bid-ask spread volatility but offer solutions to help you place smarter, more profitable trades.